Table Of Content
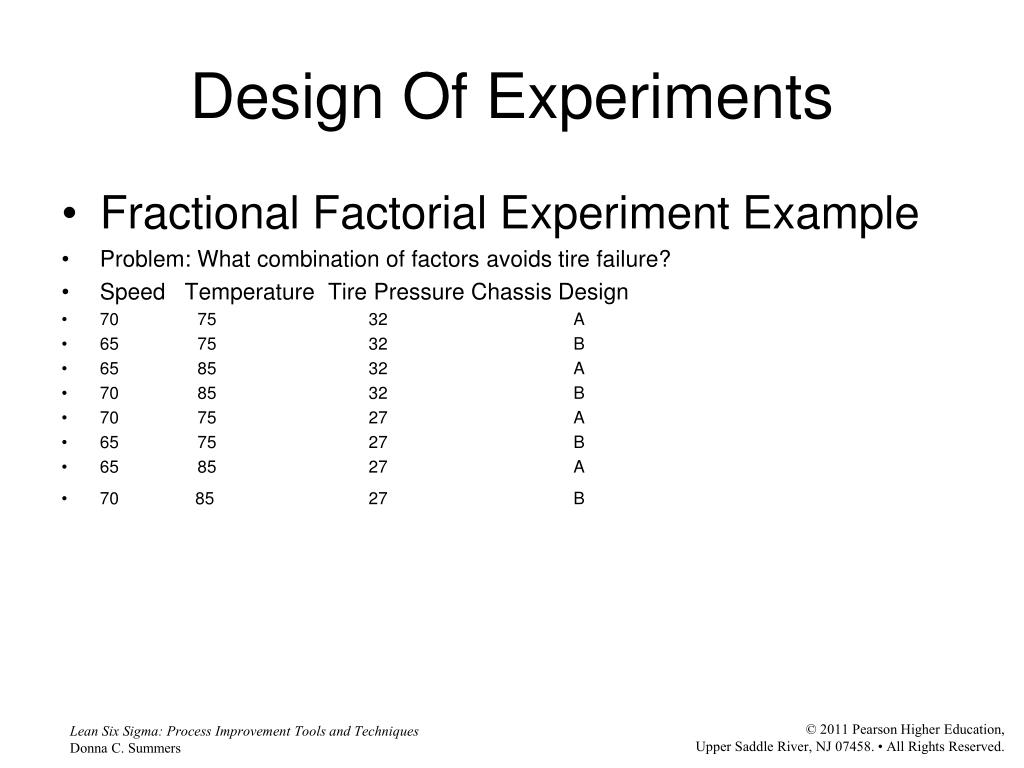
Unfortunately, most process outcomes are a function of interactions rather than pure main effects. You will need to understand the implications of that when operating your processes. When discussing the proper settings for your process variables, people often rely on what they have always done, on what Old Joe taught them years ago, or even where they feel the best setting should be.
When to use Experimental Research Design
Main concerns in experimental design include the establishment of validity, reliability, and replicability. For example, these concerns can be partially addressed by carefully choosing the independent variable, reducing the risk of measurement error, and ensuring that the documentation of the method is sufficiently detailed. Related concerns include achieving appropriate levels of statistical power and sensitivity. Of all the types, the simplest type of experimental design is the completely randomized design, in which the participants are randomly assigned to the treatment groups. The main advantage of using this method is that it avoids bias and controls the role of chance.
How many runs do I need for a full factorial DOE?
We would have missed out acquiring the optimal temperature and time settings based on our previous OFAT experiments. Say we want to determine the optimal temperature and time settings that will maximize yield through experiments. In a true experiment design, the participants of the group are randomly assigned. So, every unit has an equal chance of getting into the experimental group. In this article, we are going to discuss these different experimental designs for research with examples.
What is DOE? Design of Experiments Basics for Beginners
Below is an example of a table that shows the yield that was obtained when changing the volume from 500 to 700 ml. In the scatterplot on the right, we have plotted the measured yield against the change in reaction volume, and it doesn’t take long to see that the best volume is located at 550 ml. So, for example, first we might fix the pH at 3, and change the volume of the reaction container from a low setting of 500ml to a high of 700ml. In order to understand why Design of Experiments is so valuable, it may be helpful to take a look at what DOE helps you achieve. A good way to illustrate this is by looking at an alternative approach, one that we call the “COST” approach. It’s also helpful to see an example of the kinds of Factors that are in an Experiment.
Experimental Designs

In a factorial design, participants are randomly assigned to one of several groups, each of which receives a different combination of two or more independent variables. This paper presents the application of an advanced quality management tool, the design of experiments (DOE), in order to characterise a new material (carbon fibre-reinforced polyamide) used in the 3D printing process. The study focuses on the definition of optimal 3D printing parameters, such as nozzle size, temperature, print speed, layer height and print orientation, to achieve desired mechanical properties. The results show that layer height and print orientation have a significant effect on mechanical properties and printing time.
Statistical significance
Power and optimal study design in iPSC-based brain disease modelling Molecular Psychiatry - Nature.com
Power and optimal study design in iPSC-based brain disease modelling Molecular Psychiatry.
Posted: Wed, 16 Nov 2022 08:00:00 GMT [source]
Apart from these types of experimental design research in statistics, there are other two methods used in the research process such as randomized block design and completely randomized design. With three variables, machine speed, fill speed, and carbonation level, how many different unique combinations would you have to test to explore all the possibilities? Which combination of machine speed, fill speed, and carbonation level will give you the most consistent fill? The experimentation using all possible factor combinations is called a full factorial design. The study of the design of experiments is an important topic in metascience.
Contents
With the diagram, you see different factors such as the equipment (oven) and ingredients. In your design, you must capture the factors specifically so that, just as in any experiment, you can replicate them. If your cake burns on the bottom, is it the heating process (conventional / convection), is it the powering source (electrical or gas), and/or is it the placement of the racks (bottom, middle, top) within the oven? Or do all three aspects of the oven factor impact the interactions among other factors and your final result? You must accurately document each factor to better know how changes may shape the outcome.
Factors might include preheating the oven, baking time, ingredients, amount of moisture, baking temperature, etc.-- what else? You probably follow a recipe so there are many additional factors that control the ingredients - i.e., a mixture. What parts of the recipe did they vary to make the recipe a success?
DOE looks at different ranges within factors.
A full factorial design provides information about all the possible interactions. Fractional factorial designs will provide limited interaction information because you did not test all the possible combinations. But, what if you aren’t able to run the entire set of combinations of a full factorial? What if you have monetary or time constraints, or too many variables? This is when you might choose to run a fractional factorial, also referred to as a screening DOE, which uses only a fraction of the total runs. That fraction can be one-half, one-quarter, one-eighth, and so forth depending on the number of factors or variables.
An experimental design where treatments aren’t randomly assigned is called a quasi-experimental design. You can either use full factorial designs with all possible factor combinations, or fractional factorial designs using smaller subsets of the combinations. The use of a control group is an important experimental design method that involves having a group of participants that do not receive the treatment or intervention being studied. The control group is used as a baseline to compare the effects of the treatment group.
Project Managers who excel in planning will be able to apply that skill to the running of a Design of Experiments for their project. Specific tasks must be conducted in a certain sequence to achieve statistically relevant results. We can see three main reasons that DOE Is a better approach to experiment design than the COST approach. In this way, DOE allows you to construct a carefully prepared set of representative experiments, in which all relevant factors are varied simultaneously. The optimal combination for the best yield would be a volume of 550 ml and pH 4.5.
Experimental research design should be used when a researcher wants to establish a cause-and-effect relationship between variables. It is particularly useful when studying the impact of an intervention or treatment on a particular outcome. Inferential statistics are used to make inferences or generalizations about a larger population based on the data collected in the study. This involves dividing participants into subgroups or blocks based on specific characteristics, such as age or gender, in order to reduce the risk of confounding variables. This involves randomly assigning participants to different groups or treatments to ensure that any observed differences between groups are due to the treatment and not to other factors.
The control group tells us what would have happened to your test subjects without any experimental intervention. First, you may need to decide how widely to vary your independent variable. Start by simply listing the independent and dependent variables. As well as these savings, DOE achieves higher precision and reduced variability when estimating the effects of each factor or interaction than using OFAT. It also systematically estimates the interaction between factors, which is not possible with OFAT experiments. Design of experiments allows you to test numerous factors to determine which make the largest contributions to yield and taste.
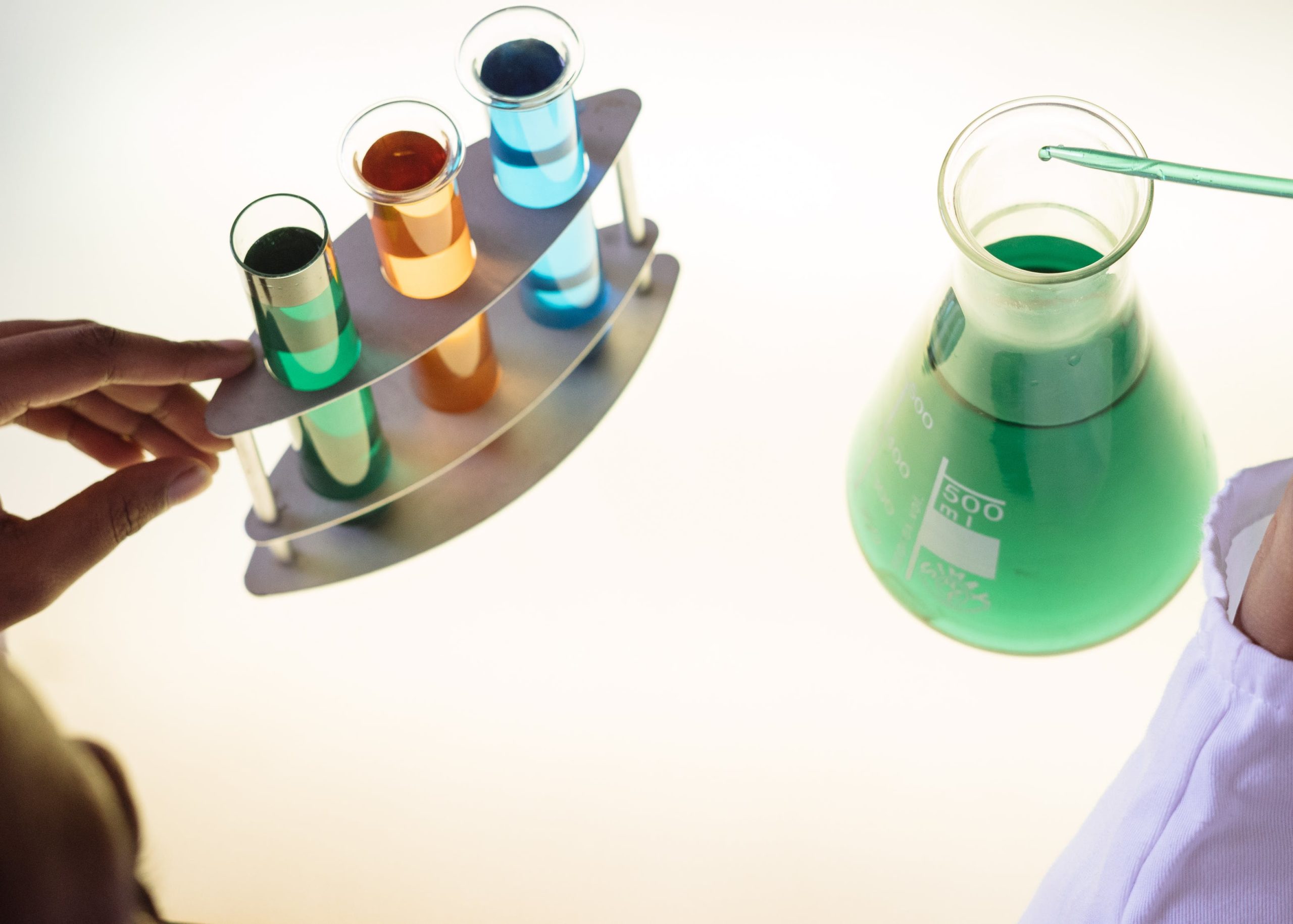
However, the focus of the course is on the design and not on the analysis. Please include what you were doing when this page came up and the Cloudflare Ray ID found at the bottom of this page.
A unique application of DOE in marketing is called conjoint analysis. A web-based company wanted to design its website to increase traffic and online sales. Doing a traditional DOE was not practical, so leadership decided to use conjoint analysis to help them design the optimal web page. Doing a designed experiment as opposed to using a trial-and-error approach has a number of benefits. You can visualize, explore your model and find the most desirable settings for your factors using the JMP Prediction Profiler.
Probably many factors, temperature and moisture, various ratios of ingredients, and presence or absence of many additives. Now, should one keep all the factors involved in the experiment at a constant level and just vary one to see what would happen? This is one of the concepts that we will address in this course. Then measure your chosen response variable at several (at least two) settings of the factor under study. If changing the factor causes the phenomenon to change, then you conclude that there is indeed a cause-and-effect relationship at work. Run all possible combinations of factor levels, in random order to average out effects of lurking variables.
No comments:
Post a Comment